Clinical Redesign
Our care delivery team designs new care delivery models that harness technology to deliver high-quality, innovative healthcare services across all environments — from traditional healthcare facilities to care at home.
These new care delivery models prioritize patient experience and patient outcomes, not volume, and are designed for and tested in value-based payment models. Our goal is to give our patients care that’s tailored to their needs, at the right time and in the appropriate setting. And by transforming how we deliver healthcare services and how we pay for them, we fundamentally shift the incentives and deliver better care for a lower cost.
Featured care delivery:
Lung Nodule Follow-up Care
Our team developed an innovative approach using natural language processing (NLP) to identify patients with a positive lung nodule who did not have documentation of follow-up.
NLP was used for text analysis of radiology reports and extraction of key words to detect the presence of nodules, classify characteristics such as size and number of nodules, and parse out follow-up recommendations. These data were then combined with Epic documentation to determine whether follow-up recommendations were completed — a result that was achieved with 84 percent accuracy.
There were 1,864 patients with no documentation of follow-up. These patients were further categorized and prioritized for follow-up based on the size of the nodule.
Our Care Gaps team initiated a 3-month pilot that included outreach to the prioritized patients to notify them of the findings, confirm status of follow-up and enroll them in the System to Track Abnormalities of Importance Reliably (STAIR) program as appropriate.
The STAIR program established a care plan for each patient and made sure recommended follow-up was completed. At the end of the pilot, 58 percent were being followed up by their primary care provider (PCP) or specialist, 13 percent had completed the STAIR program, 11 percent were in the outreach process and 3.5 percent declined follow-up. In 3.5 percent of cases, no lung nodule was found upon chart review.
Overall, 89 percent of the care gaps were closed.
Chronic Disease Management Command Center
Our teams are developing an innovative approach for people struggling with chronic disease to make better health a reality. The Chronic Disease Management Command Center is a care model designed to improve outcomes and lower healthcare costs for those living with chronic disease or diseases. The Command Center will harness groundbreaking technology, including remote patient monitoring, patient reported outcomes, virtual visits and artificial intelligence to transform the way our care management infrastructure interacts with those we care for in our hospitals and clinics. By focusing on personalized holistic experiences and interventions, we can empower those with chronic disease to take a more active role in their care and ultimately live healthier lives.
Our initial focus will be on five chronic conditions:
- Diabetes
- Chronic obstructive pulmonary disease (COPD)
- Congestive heart failure
- Stroke
- Hypertension
We plan to add additional conditions as the platform grows and scale our efforts across the entire Geisinger system by 2021.
Predicting Stroke Risk
Partnering with Tempus, a technology company, we’re using artificial intelligence (AI) to predict patients’ risk of new atrial fibrillation (AF) and AF-related stroke. This allows our clinicians to act earlier, which can improve patient outcomes.
The study, published in Circulation, used electrical signals from the heart — measured from a 12-lead electrocardiogram (ECG) — to identify patients who are likely to develop AF, including those at risk for AF-related stroke.
Our team of data scientists and medical researchers used 1.6 million ECGs from 430,000 patients over 35 years of patient care at Geisinger. These data were used to train a deep neural network — a specialized class of artificial intelligence — to predict, among patients without a previous history of AF, who would develop AF within 12 months. The neural network performance exceeded that of current clinical models for predicting AF risk. Furthermore, 62 percent of patients without known AF who experienced an AF-related stroke within three years were identified as high risk by the model before the stroke occurred.
Knowing that the high risk prediction precedes many AF-related strokes, we can change the way patients are screened and treated, potentially preventing severe outcomes.
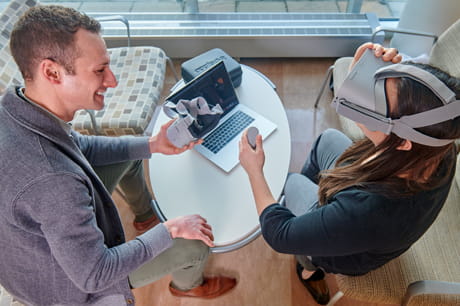